What does EBL mean in UNCLASSIFIED
EBL, or Explanation-Based Learning, is a type of machine learning that uses previously acquired knowledge to generate new rules and understandings from a set of data. It is widely used in fields such as Artificial Intelligence (AI), Information Technology (IT) and Management Information Systems (MIS) to provide better insight into complex problems. The goal of EBL is to help an artificial system come up with solutions faster and more efficiently than it would by simply crunching through data.
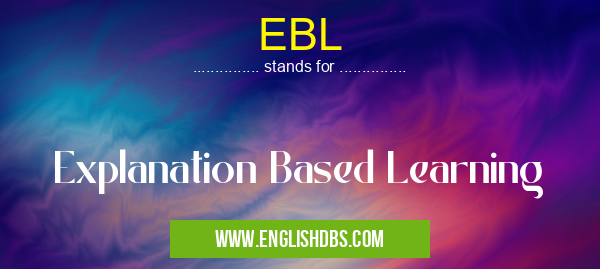
EBL meaning in Unclassified in Miscellaneous
EBL mostly used in an acronym Unclassified in Category Miscellaneous that means Explanation Based Learning
Shorthand: EBL,
Full Form: Explanation Based Learning
For more information of "Explanation Based Learning", see the section below.
Definition
EBL stands for Explanation-Based Learning. This technique of machine learning uses prior knowledge, patterns, and inferences to come up with theories and decisions from a given dataset. It works by taking the raw data, analyzing it against existing knowledge, making conclusions based on that analysis, then using those conclusions to form new hypotheses about the data.
Process of EBL
EBL works as a type of supervised learning approach where information collected from previous experiences are used to solve new problems quickly. The process begins with the collection of facts related to the problem being studied; these facts are used as input for the explanation-based learning algorithm which can then create rules that explain the behavior observed within the facts gathered. After this has been done, an inference engine can be used on this established set of rules to draw out conclusions on potential outcomes based on different circumstances or values given.
Advantages
One major advantage of Explanation-Based Learning is its speed when compared with other Machine Learning methods since much less training time is required due to its reliance on existing knowledge bases. Additionally, because of its use of inferences and rules derived from known facts, EBL can be applied in situations where traditional ML algorithms may not work effectively or efficiently enough due to small datasets or incomplete information - allowing for more comprehensive analysis than what may otherwise be possible. Finally, because EBL relies heavily on rule-matching algorithms instead of solely relying on brute force approaches means it uses less computational power while also providing better accuracy than other Machine Learning approaches in most cases.
Essential Questions and Answers on Explanation Based Learning in "MISCELLANEOUS»UNFILED"
What is Explanation Based Learning?
Explanation Based Learning (EBL) is a type of artificial intelligence technique used to induce general rules from specific examples. It works by building a model of problem solving techniques and strategies from an initial set of data to create new concepts. EBL can be used to solve problems in fields such as robotics, natural language processing, computer vision, and automated planning.
How does Explanation Based Learning differ from traditional AI techniques?
Explanation Based Learning is distinct from traditional Artificial Intelligence techniques because it focuses on creating general solutions from specific data. Rather than simply finding the best solution to a given problem, EBL seeks to find all possible solutions and then use those solutions to build a deeper understanding of the problem.
What are the benefits of using Explanation Based Learning?
The main benefit of using EBL is its ability to develop models that can solve complex problems with greater creativity and accuracy than most traditional Artificial Intelligence techniques. Additionally, once an initial set of data has been provided, there is less need for manual input which makes it more efficient in terms of time and resources.
Are there any potential drawbacks with Explanation Based Learning?
One potential drawback with EBL is that it may require more computational power than many traditional AI techniques due to the complexity of the models it requires. Additionally, since it can take more time to reach a solution than traditional AI methods, there may be significant delays when facing certain situations or challenges that don’t fit within its existing framework.
How does one design an effective Explanation Based Learning algorithm?
Designing an effective Explanation Based Learning Algorithm involves several key steps including data selection, preprocessing, feature extraction and selection along with clustering analysis and rule induction techniques. It also requires careful tuning of parameters such as learning rate and regularization methods in order to ensure accurate results.
What kind of data can be used for Explanation Based Learning algorithms?
Data used for EBL algorithms must be labeled correctly in order for them to learn effectively. Typically this means that each piece of data must have associated labels describing what category or class they belong to as well as other relevant information such as timestamps or coordinates.
Is it possible for an Explanation Based Learning system to learn without being supervised by humans?
Yes, it is possible for an EBL system to learn without being supervised by humans but this depends on the type and quality of training data available which will affect how well the system performs overall.
What are some applications where Explanation Based Learning might be useful?
There are numerous applications where EBL could be useful ranging from medical diagnosis systems that can diagnose diseases based on individual patient information; robotic navigation systems capable of autonomously navigating their environment; autonomous cars able to efficiently drive themselves; natural language processing systems capable of understanding human speech; computer vision systems capable quickly recognizing objects in images; automated planning systems able make decisions based on complex sets conditions; etc.
Final Words:
Explanation-Based Learning (EBL) provides an efficient way for machines to learn from their experience without having to go through large amounts of trial and error like traditional ML methods do. With its ability to quickly create relevant rules and inferences from known information combined with its scalability even under limited datasets make it one of the best choices when dealing with complex problems in fields such as Artificial Intelligence (AI), IT & MIS departments today – offering higher accuracy and speed than traditional Machine Learning methods while also requiring less computing resources at lower cost.
EBL also stands for: |
|
All stands for EBL |