What does SDE mean in UNCLASSIFIED
Stochastic Differential Equations (SDEs) are mathematical equations that link changes in random variables over time. SDEs are widely used in finance, physics, biology and related fields. They provide a powerful tool to simulate the dynamics of real-world processes, such as climate change and stock prices. In this article, we will discuss what SDEs mean and what they can be used for.
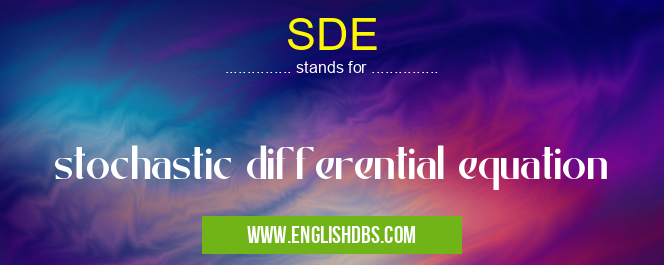
SDE meaning in Unclassified in Miscellaneous
SDE mostly used in an acronym Unclassified in Category Miscellaneous that means stochastic differential equation
Shorthand: SDE,
Full Form: stochastic differential equation
For more information of "stochastic differential equation", see the section below.
Definition
A Stochastic Differential Equation (SDE) is an ordinary differential equation with one or more non-deterministic derivative terms. It is an integral equation that links changes in a random variable over time with respect to known parameters or influences from other random variables. The standard form of an SDE is dx = f(t)dt + g(t)dW where x is a random variable, W is a random process called Brownian motion and f(t) and g(t) are functions of t (time).
Application Areas
The practical application areas of SDEs include financial modeling, image processing, environmental sciences, robotics and control system design. For instance, the Black-Scholes model for valuing option contracts on stocks relies heavily on SDEs. In finance, SDEs can be used to calculate volatility in stock prices and option premiums. In environmental sciences, stochastic models can help researchers understand the spread of pollutants across time and space more accurately. In robotics applications such as control systems design, stochastic analysis can help distinguish between noise in measurement data or uncertain behaviors from other components of the system.
Advantages
SDEs have several advantages over deterministic differential equations due to their inherent randomness nature. Unlike deterministic equations where slight errors or discrepancies may cause solutions to be incorrect or misleading, SDEs account for unavoidable uncertainty which helps them capture more realistic scenarios than those obtained with deterministic models. Moreover, since the state of the system at any point in time is unpredictable due to its random nature, it allows us to use less data when constructing simulations or analysis compared to deterministic approaches - rushing us greater computational efficiency if needed.
Limitations
Although powerful tools for simulating real-world processes with high accuracy and speeds approaching those found in deterministic models, SDEs have some notable limitations that should be taken into account when used for certain tasks. Since they rely on assumptions about underlying distributions (for example normal distributions), results derived from these models may not reflect true behavior under different situations outside their assumed assumptions - leading potential inaccuracies depending on how far removed our actual situation is from those assumptions made at the beginning of our work with these models. Additionally due to their inherent randomness nature there may be difficulty deciding how long a simulation run should last in order for valid results to be obtained forcing us to perhaps make conservative estimates which could lead possible discrepancies when compared against real world outcomes if we were lucky enough to obtain them directly without having relied upon simulations first hand.
Essential Questions and Answers on stochastic differential equation in "MISCELLANEOUS»UNFILED"
What is a Stochastic Differential Equation (SDE)?
A Stochastic Differential Equation (SDE) is a differential equation with random variables or stochastic processes included in the formulation. These equations are used to describe natural phenomena that contain an element of randomness and can be used to model financial markets and other types of uncertainty.
How does an SDE differ from a regular differential equation?
Regular differential equations are deterministic, meaning they use constants or known functions as inputs instead of random variables. SDEs take into account factors such as noise and volatility, making them relevant for certain problems that require more complex and stochastic solutions.
How is an SDE solved?
SDEs are usually solved using numerical methods such as Monte Carlo simulations, finite difference methods, or variance reduction techniques. In some cases, analytical solutions can also be obtained via transformation of the SDE into simpler forms that can be analytically solved.
What is the Euler-Maruyama Method?
The Euler-Maruyama Method is a numerical method used to approximate solutions to stochastic differential equations. It uses a discretization technique in order to generate the numerical solution, where small time steps are taken and then combined together to give an approximation of the solution at any point in time.
What applications can use SDEs?
Stochastic Differential Equations have many applications including finance, robotics, control theory, thermodynamics, actuarial science and engineering design. They can also be used for modelling complex systems with uncertain parameters such as weather forecasting and portfolio optimization.
How do you represent SDEs mathematically?
A stochastic differential equation is typically written as dX = f(X) dt + g(X) dB(t), which describes the evolution of X over time given some function f(X) for deterministic behavior and another function g(X) for random variable values. Here B(t) denotes Brownian motion which captures the uncertainty associated with X's evolution over time.
Why are SDEs important in finance?
In finance models it is often assumed that all prices follow a random walk - meaning that future prices are unknown but partially determined by past prices – so solving for these models requires stochastic processes described by SDEs in order to capture these nuances accurately.
Can you solve nonlinear SDEs?
Yes - nonlinear stochastic differential equations can be solved numerically using various iterative techniques such as Taylor series expansions or methods specific to nonlinear cases such as Runge-Kutta-type algorithms. Alternatively, there exist analytic approaches which may reduce complex problems into solvable forms such as Freidlin-Wentzell theory or Girsanov's theorem for change of measure transformations within diffusion processes.
SDE also stands for: |
|
All stands for SDE |